Research Activities > Programs >
Sparse Representation in Redundant Systems
>
David Donoho
|

CSIC Building (#406),
Seminar Room 4122.
Directions: home.cscamm.umd.edu/directions
|
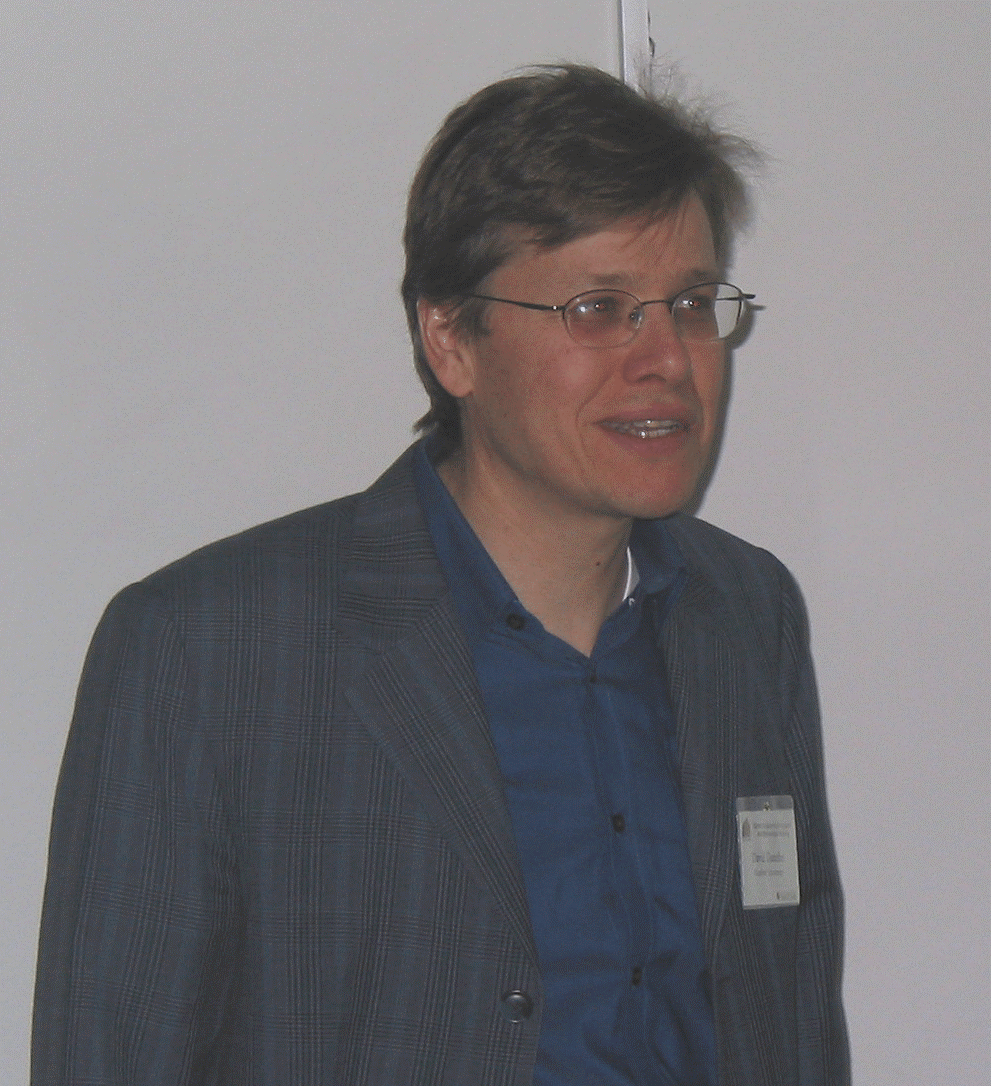
The Sparsity Phase Transition
Dr. David Donoho
Statistics at Stanford University
|
Abstract:
For most large d × n matrices A
there is a sparsity threshold EBP(A)
so that for every system of linear equations y=Ax with at most k nonzeros, k <
EBP(A), the sparsest solution is the solution with minimal
l1 norm. Recent work has exhibited a function ρN(δ)
> 0 so that EBP(A) ≥ ρN(d/n)
d, and computed it numerically. This function is surprisingly large, meaning that for `most' matrices surprisingly weak conditions on
scarcity guarantee that the l1 solution is the sparsest solution. A simple corollary is the ubiquity (for large
n) of (n,n/4,n/9) codes which are decodable by linear programming. Another simple corollary is that for most large systems
n × sqrt(2)n systems which permit a solution with fewer than .49n
nonzeros, the minimum l1 solution is the sparsest solution. In case we are interested only in nonnegative solutions, the results are even
more favorable, thus for most large systems n × 2n systems which permit a solution with fewer than .55n nonzeros, the minimum-sum nonnegative solution is the sparsest solution. Interesting ideas behind these results include neighborliness of polytopes and grassmann angles in high dimensions
ided
|
|
|